Subset SWOT data from AVISO’s FTP Server
This notebook explains how to select and retrieve SWOT LR half orbits from AVISO’s FTP Server, and subset data with a geographical area.
Files available through FTP follow the same file tree as in the THREDDS Data Server.
Data |
FTP starting point |
Browsing files URL |
---|---|---|
Swot LR L2 |
/swot_products/l2_karin/l2_lr_ssh |
https://tds.aviso.altimetry.fr/thredds/catalog/dataset-l2-swot-karin-lr-ssh-validated/catalog.html |
Swot LR L3 |
/swot_products/l3_karin_nadir/l3_lr_ssh |
Warning
To subset L2 LR SSH Unsmoothed data, which is stored as grouped Netcdf files, please consult this tutorial.
Tutorial Objectives
Download files through FTP, with selection by cycle and pass numbers
Subset data with geographical selection
Import + code
[1]:
import warnings
warnings.filterwarnings('ignore')
[2]:
import os
from getpass import getpass
import ftplib
import xarray as xr
[3]:
import cartopy.crs as ccrs
import matplotlib.pyplot as plt
[4]:
def _download_file(ftp:str, filename:str, target_directory:str):
print(f"Download file: {filename}")
try:
local_filepath = os.path.join(target_directory, filename)
with open(local_filepath, 'wb') as file:
ftp.retrbinary('RETR %s' % filename, file.write)
return local_filepath
except Exception as e:
print(f"Error downloading {filename}: {e}")
def _get_last_version_filename(filenames):
versions = {int(f[-5:-3]): f for f in filenames}
return versions[max(versions.keys())]
def _select_filename(filenames, only_last):
if not only_last: return filenames
return [_get_last_version_filename(filenames)]
def ftp_download_files(ftp_path, level, variant, cycle_numbers, half_orbits, output_dir, only_last=True):
""" Download half orbits files from AVISO's FTP Server.
Args:
ftp_path
path of the FTP fileset
level
L2 or L3
variant
Basic, Expert, WindWave or Unsmoothed
cycle_numbers
list of cycles numbers
half_orbits
list of passes numbers
output_dir
output directory
only_last
if True (Default), downloads only the last version of a file if several versions exist.
Downloads all versions otherwise.
Returns:
The list of local files.
"""
# Set up FTP server details
ftpAVISO = 'ftp-access.aviso.altimetry.fr'
try:
# Logging into FTP server using provided credentials
with ftplib.FTP(ftpAVISO) as ftp:
ftp.login(username, password)
ftp.cwd(ftp_path)
print(f"Connection Established {ftp.getwelcome()}")
downloaded_files = []
for cycle in cycle_numbers:
cycle_str = '{:03d}'.format(cycle)
cycle_dir = f'cycle_{cycle_str}'
print(ftp_path+cycle_dir)
ftp.cwd(cycle_dir)
for half_orbit in half_orbits:
half_orbit_str = '{:03d}'.format(half_orbit)
pattern = f'SWOT_{level}_LR_SSH_{variant}_{cycle_str}_{half_orbit_str}'
filenames = []
try:
filenames = ftp.nlst(f'{pattern}_*')
# No version in L3 filenames
if level=="L3": only_last=False
filenames = _select_filename(filenames, only_last)
except Exception as e:
print(f"No pass {half_orbit}")
local_files = [_download_file(ftp, f, output_dir) for f in filenames]
downloaded_files += local_files
ftp.cwd('../')
return downloaded_files
except ftplib.error_perm as e:
print(f"FTP error: {e}")
except Exception as e:
print(f"Error: {e}")
def _normalized_ds(ds, lon_min, lon_max):
lon = ds.longitude.values
lon[lon < lon_min] += 360
lon[lon > lon_max] -= 360
ds.longitude.values = lon
return ds
def _subset_ds(file, variables, lon_range, lat_range, output_dir):
print(f"Subset dataset: {file}")
swot_ds = xr.open_dataset(file)
swot_ds = swot_ds[variables]
swot_ds.load()
ds = _normalized_ds(swot_ds.copy(), -180, 180)
mask = (
(ds.longitude <= lon_range[1])
& (ds.longitude >= lon_range[0])
& (ds.latitude <= lat_range[1])
& (ds.latitude >= lat_range[0])
).compute()
swot_ds_area = swot_ds.where(mask, drop=True)
if swot_ds_area.sizes['num_lines'] == 0:
print(f'Dataset {file} not matching geographical area.')
return None
for var in list(swot_ds_area.keys()):
swot_ds_area[var].encoding = {'zlib':True, 'complevel':5}
filename = "subset_"+file[10:]
print(f"Store subset: {filename}")
filepath = os.path.join(output_dir, filename)
swot_ds_area.to_netcdf(filepath)
return filepath
def subset_files(filenames, variables, lon_range, lat_range, output_dir):
""" Subset datasets with geographical area.
Args:
filenames
the filenames of datasets to subset
variables
variables to select
lon_range
the longitude range
lat_range
the latitude range
output_dir
output directory
Returns:
The list of subsets files.
"""
return [subset_file for subset_file in [_subset_ds(f, variables, lon_range, lat_range, output_dir) for f in filenames] if subset_file is not None]
def plot_datasets(filenames, variable, extent=None):
cb_args = dict(
add_colorbar=True,
cbar_kwargs={"shrink": 0.3}
)
plot_kwargs = dict(
x="longitude",
y="latitude",
cmap="Spectral_r",
vmin=-0.2,
vmax=0.2,
)
fig, ax = plt.subplots(figsize=(12, 8), subplot_kw=dict(projection=ccrs.PlateCarree()))
if extent: ax.set_extent(extent)
for filename in filenames:
ds = xr.open_dataset(filename)
ds[variable].plot.pcolormesh(
ax=ax,
**plot_kwargs,
**cb_args)
cb_args=dict(add_colorbar=False)
ax.coastlines()
ax.gridlines(draw_labels=True)
Parameters
Define existing output folder to save results
[5]:
output_dir = "downloads"
Authentication parameters
Enter your AVISO+ credentials
[21]:
username = input("Enter username:")
Enter username: aviso-swot@altimetry.fr
[22]:
password = getpass(f"Enter password for {username}:")
Enter password for aviso-swot@altimetry.fr: ········
Data parameters
Define a geographical area
[8]:
# Mediterranean sea
lat_range = 25, 50
lon_range = -15, 40
localbox = [lon_range[0], lon_range[1], lat_range[0], lat_range[1]]
localbox
[8]:
[-15, 40, 25, 50]
Define the FTP filepath
[9]:
ftp_path = '/swot_products/l3_karin_nadir/l3_lr_ssh/v1_0_2/Expert/'
# For selecting files with a regex pattern
# Basic, Expert, WindWave, Unsmoothed
variant = "Expert"
# L2, L3
level = "L3"
Define variables to select
[10]:
variables = ['time', 'ssha']
Define data parameters
Note
Passes matching a geographical area and period can be found using this tutorial
[11]:
# Define cycles and half_orbits numbers to download
cycle_numbers = [19]
pass_numbers = [1, 2, 29, 70, 98, 236, 376]
Download files through FTP
[15]:
downloaded_files = ftp_download_files(ftp_path, level, variant, cycle_numbers, pass_numbers, output_dir, only_last=True)
Connection Established 220 192.168.10.119 FTP server ready
/swot_products/l3_karin_nadir/l3_lr_ssh/v1_0_2/Expert/cycle_019
Download file: SWOT_L3_LR_SSH_Expert_019_001_20240730T190512_20240730T195638_v1.0.2.nc
Download file: SWOT_L3_LR_SSH_Expert_019_002_20240730T195639_20240730T204805_v1.0.2.nc
Download file: SWOT_L3_LR_SSH_Expert_019_029_20240731T190543_20240731T195709_v1.0.2.nc
Download file: SWOT_L3_LR_SSH_Expert_019_070_20240802T061503_20240802T070629_v1.0.2.nc
Download file: SWOT_L3_LR_SSH_Expert_019_098_20240803T061534_20240803T070700_v1.0.2.nc
Download file: SWOT_L3_LR_SSH_Expert_019_236_20240808T043516_20240808T052642_v1.0.2.nc
Download file: SWOT_L3_LR_SSH_Expert_019_376_20240813T043752_20240813T052918_v1.0.2.nc
[16]:
downloaded_files
[16]:
['downloads/SWOT_L3_LR_SSH_Expert_019_001_20240730T190512_20240730T195638_v1.0.2.nc',
'downloads/SWOT_L3_LR_SSH_Expert_019_002_20240730T195639_20240730T204805_v1.0.2.nc',
'downloads/SWOT_L3_LR_SSH_Expert_019_029_20240731T190543_20240731T195709_v1.0.2.nc',
'downloads/SWOT_L3_LR_SSH_Expert_019_070_20240802T061503_20240802T070629_v1.0.2.nc',
'downloads/SWOT_L3_LR_SSH_Expert_019_098_20240803T061534_20240803T070700_v1.0.2.nc',
'downloads/SWOT_L3_LR_SSH_Expert_019_236_20240808T043516_20240808T052642_v1.0.2.nc',
'downloads/SWOT_L3_LR_SSH_Expert_019_376_20240813T043752_20240813T052918_v1.0.2.nc']
Plot downloaded half orbits
[17]:
plot_datasets(downloaded_files, 'ssha', extent=[-180, 180, -80, 80])
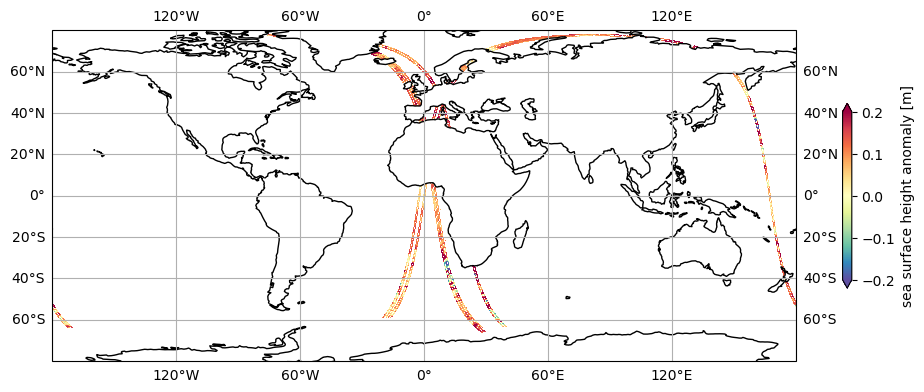
Subset data
[18]:
subset_filenames = subset_files(downloaded_files, variables, lon_range, lat_range, output_dir)
Subset dataset: downloads/SWOT_L3_LR_SSH_Expert_019_001_20240730T190512_20240730T195638_v1.0.2.nc
Store subset: subset_SWOT_L3_LR_SSH_Expert_019_001_20240730T190512_20240730T195638_v1.0.2.nc
Subset dataset: downloads/SWOT_L3_LR_SSH_Expert_019_002_20240730T195639_20240730T204805_v1.0.2.nc
Dataset downloads/SWOT_L3_LR_SSH_Expert_019_002_20240730T195639_20240730T204805_v1.0.2.nc not matching geographical area.
Subset dataset: downloads/SWOT_L3_LR_SSH_Expert_019_029_20240731T190543_20240731T195709_v1.0.2.nc
Store subset: subset_SWOT_L3_LR_SSH_Expert_019_029_20240731T190543_20240731T195709_v1.0.2.nc
Subset dataset: downloads/SWOT_L3_LR_SSH_Expert_019_070_20240802T061503_20240802T070629_v1.0.2.nc
Store subset: subset_SWOT_L3_LR_SSH_Expert_019_070_20240802T061503_20240802T070629_v1.0.2.nc
Subset dataset: downloads/SWOT_L3_LR_SSH_Expert_019_098_20240803T061534_20240803T070700_v1.0.2.nc
Store subset: subset_SWOT_L3_LR_SSH_Expert_019_098_20240803T061534_20240803T070700_v1.0.2.nc
Subset dataset: downloads/SWOT_L3_LR_SSH_Expert_019_236_20240808T043516_20240808T052642_v1.0.2.nc
Store subset: subset_SWOT_L3_LR_SSH_Expert_019_236_20240808T043516_20240808T052642_v1.0.2.nc
Subset dataset: downloads/SWOT_L3_LR_SSH_Expert_019_376_20240813T043752_20240813T052918_v1.0.2.nc
Store subset: subset_SWOT_L3_LR_SSH_Expert_019_376_20240813T043752_20240813T052918_v1.0.2.nc
[19]:
subset_filenames
[19]:
['downloads/subset_SWOT_L3_LR_SSH_Expert_019_001_20240730T190512_20240730T195638_v1.0.2.nc',
'downloads/subset_SWOT_L3_LR_SSH_Expert_019_029_20240731T190543_20240731T195709_v1.0.2.nc',
'downloads/subset_SWOT_L3_LR_SSH_Expert_019_070_20240802T061503_20240802T070629_v1.0.2.nc',
'downloads/subset_SWOT_L3_LR_SSH_Expert_019_098_20240803T061534_20240803T070700_v1.0.2.nc',
'downloads/subset_SWOT_L3_LR_SSH_Expert_019_236_20240808T043516_20240808T052642_v1.0.2.nc',
'downloads/subset_SWOT_L3_LR_SSH_Expert_019_376_20240813T043752_20240813T052918_v1.0.2.nc']
Plot subsets
[20]:
plot_datasets(subset_filenames, 'ssha', extent=localbox)
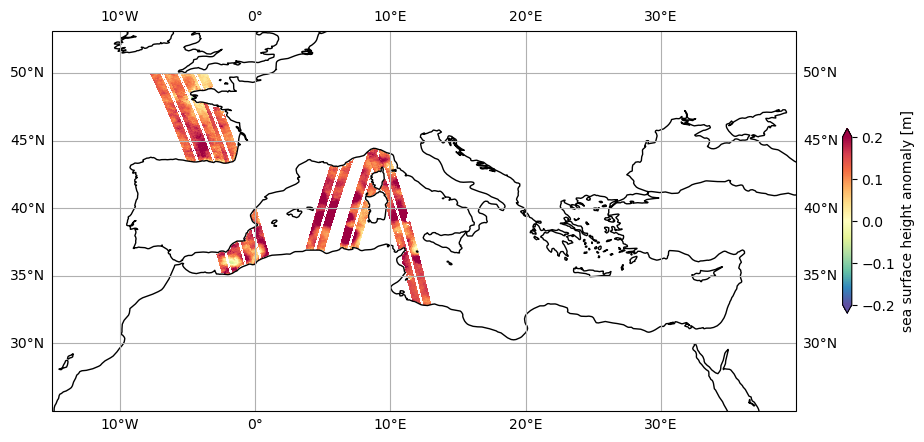